The main goal of this project was to conduct a state-of-the-art analysis of the evolution of operational concepts and technologies in order to develop and maintain a functional roadmap for each operational use case.
The TAPAS project particularly focused on researching conflict detection and resolution (CDR) in the tactical phase, air traffic flow management (ATFCM) in the pre-tactical phase, and dynamic airspace configuration.
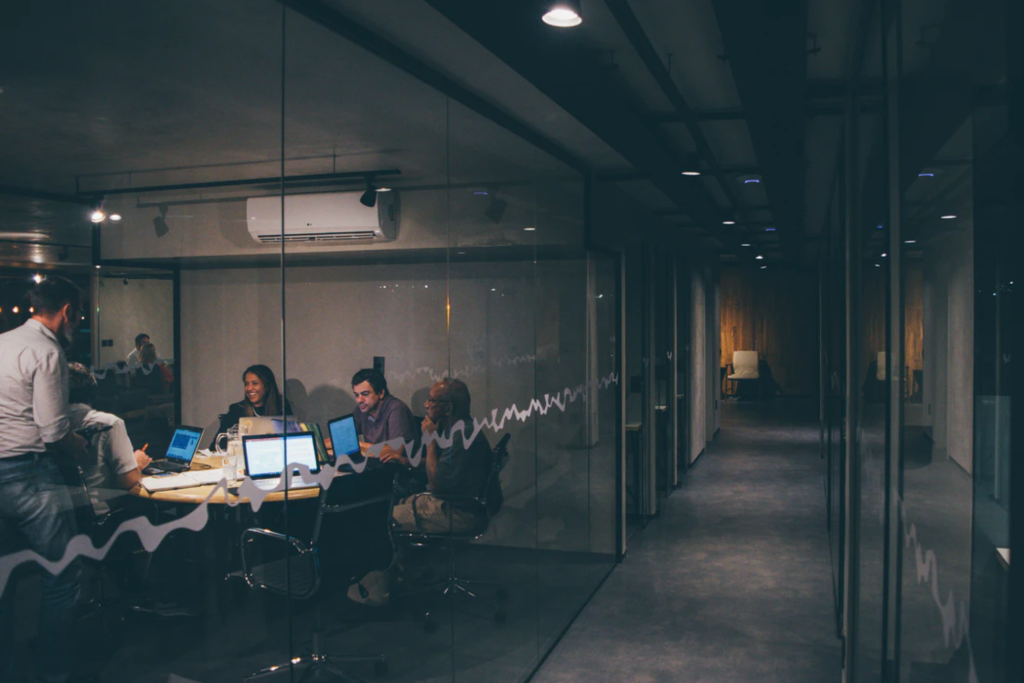
To achieve this, TAPAS employed eXplainable Artificial Intelligence (XAI) techniques alongside Visual Analytics, which helped to explore the trade-offs between the performance of AI implementation and its suitability for deployment in certain applications in terms of explainability. Not all use cases have similar requirements: an air traffic controller will need a deeper understanding of solutions provided by an AI-based function than an operator in a critical safety scenario. Not all scenarios are explained; they had to be investigated in the TAPAS research.
TAPAS aimed to provide clear and useful results that facilitate the introduction and implementation of AI applications in Air Traffic Management (ATM) systems, particularly those related to automation levels 2 and 3, as outlined in the central ATM plan and the airspace architecture study that ATM will address over the next 10 years.